Real-time panoramic depth maps using convolutional neural networks
Po Kong Lai, Shuang Xie, Jochen Lang and Robert Laganière University of Ottawa Ottawa, ON, Canada |
|
---|---|
Questions? Drop us a line |
Overview
Stereo panoramic images and videos are popular media formats for displaying content captured from visual sensors through virtual reality (VR) headsets. By displaying a different panoramic image for each eye, 3D human vision can be simulated and the viewer is allowed rotational freedom but not positional freedom. In order for positional freedom to be achieved a depth map is required. In this work we propose an approach which utilizes convolutional neural networks (CNNs) to directly convert a pair of stereo panoramic images into panoramic depth map. This depth map allows for true 3D videos with 6 degrees of freedom (DoF) viewing in a VR headset.
Training data examples
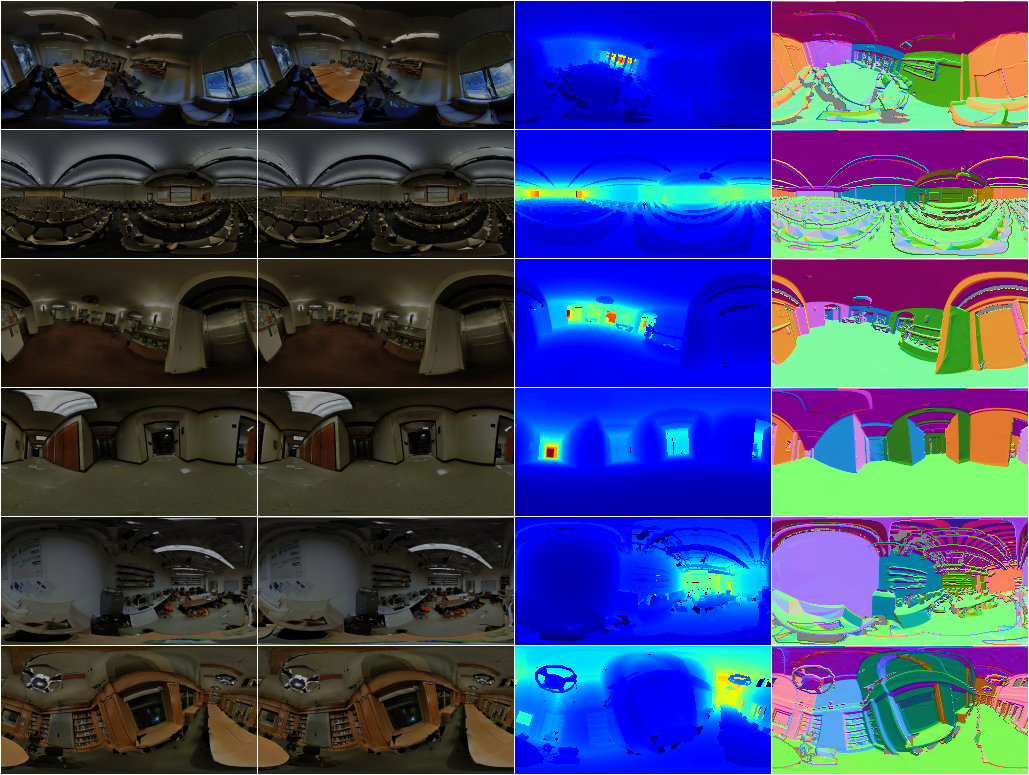
Example output
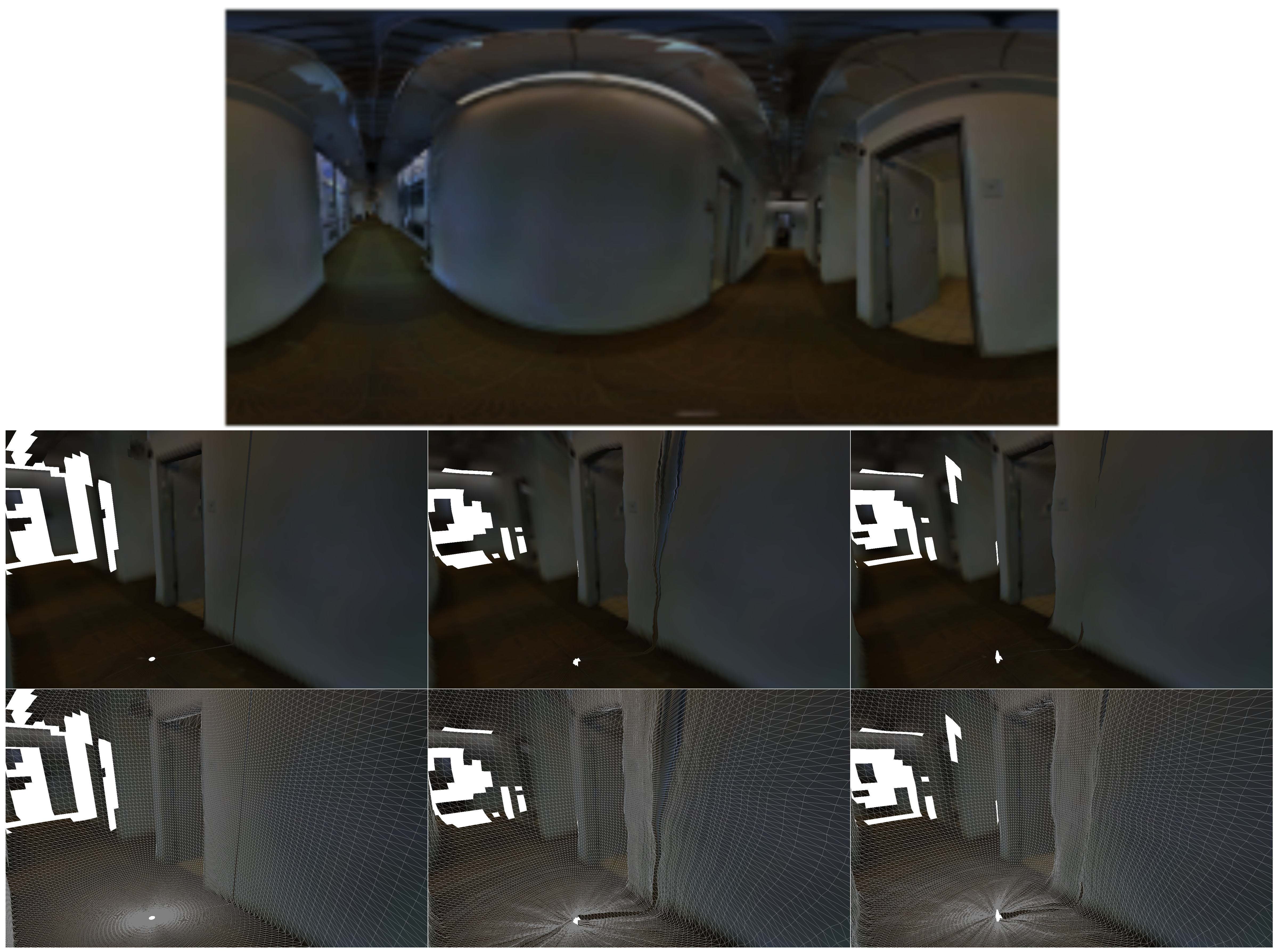
Meshed visualizations of the ground truth (left), our appproach without stereo panoramic loss (center) and with stereo panoramic loss (right). The top image is one of the stereo paranomic color images used as input.